
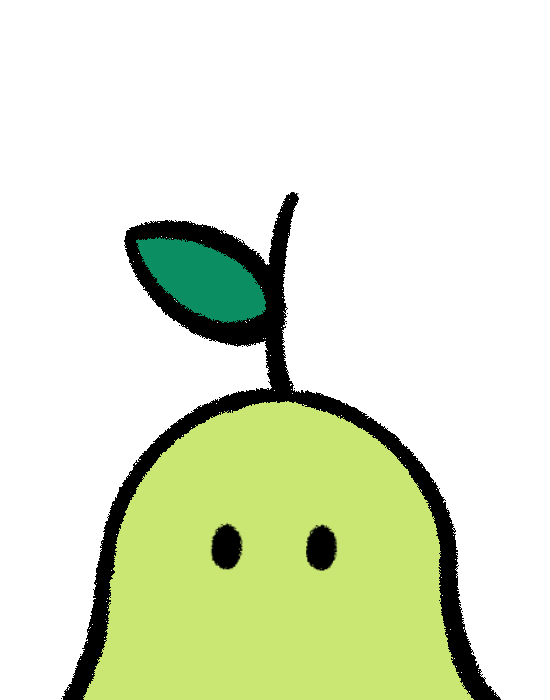
Planning Matters
If you've picked up a book on statistics, you've probably come across the idea of a research hypothesis. It’s one of the most foundational ideas in all science. It’s also more complex than an “if-this, then-that” statement. A research hypothesis is a testable, measurable prediction between two or more variables. If this variable changes in this way, then you expect this type of measurable change in the other variable. But why care about hypotheses?
Forming a hypothesis by yourself or with your stakeholders is possibly the most fruitful thing you can do when designing quantitative studies. The hypothesis is great to help you specify and clearly define the things your measurement instrument needs to measure and record from every person that interacts with it.
Your instrument can only ever measure and record some, specific quantitative data. You have to be conscious and selective about how your instrument will work. For example, you’ll never know where people buy ice cream if you don’t ask them in your survey. You can't magically know or add this extra data after you are done collecting data.
You write a hypothesis to help focus and narrow your attention, essentially saying that these are the things that your instrument has to be on the lookout for. It must collect this specific data to help us look at this specific hypothesis and determine if there's clear evidence to support or refute it. You can see examples of hypotheses here or in the guide below.
Guide 06: A Research Hypothesis Checklist
You can write different types of hypotheses, making it more likely you create your instrument in a way that’s effective and useful.
Different Kinds of Hypotheses
Quantitative research uses deductive reasoning. Deductive reasoning means you're looking for evidence for whatever pattern or relationship you’re expecting to find. The initial assumption that quantitative research takes is that your expectations are wrong. The world is simple. There aren't any real, small, changing, or hidden relationships among the variables you set to study.
You, the researcher, however, think the opposite. You plan quantitative studies built around testable hypotheses because you expect or believe that people and their world is, in fact, more complex than assumed. You set out to find evidence to disprove this idea of a simple, static (or boring) world.
Every time you plan to use hypotheses in your quantitative studies, you actually write two research hypotheses. What’s interesting is you write one hypothesis first and then work backward to write the opposite for the second.
The null hypothesis (abbreviated H0) is what you write second. The null represents the status quo or the everyday normal. The null is assumed to be true unless there's strong evidence to suggest otherwise (think innocent until proven guilty).
What’s interesting is that you write the alternate hypothesis (abbreviated HA) first. The alternate represents a possible change to regular, everyday static thinking. You write the alternate hypothesis first because you’re designing your quantitative study around it.
You think the null is false, so you set out to find evidence against it. If you find that evidence, you can infer that your alternate might be true. When you test your hypotheses, you’re trying to do one of two things: use evidence to reject the null hypothesis (Ho) in favor of the alternate (Ha), or you fail to reject the null hypothesis.
Note that there’s a subtle distinction in the words used here. When you don’t have enough evidence against the null, you’re not inferring that the null is true. You’re saying that there was or wasn’t enough evidence (in the form of your quantitative data) to not to reject the null. The null hypothesis could be in fact be false, but your study didn’t produce any data to challenge it.
You might use a statistical method to interpret your quantitative study results to test your hypothesis. Based on the significance of the test results, you might conclude you have enough information against the null hypothesis (statistical methods and tests are covered more in this Topic).
No matter what hypotheses you write, the act of specifying what to study can quickly increase not only your focus but your confidence when interpreting your quantitative findings. But this interpretation is tricky: no matter how much evidence you have, you can’t accept you alternate hypothesis.
Hypothesis Not Accepted
A quick note: the language used in null hypothesis significance testing (NHST) is not only hard to understand but inconsistent. You can see different language used when you read about hypotheses online. This confusing wording has made it easy for researchers and non-researchers to make mistakes or come to incorrect conclusions. This section tries to simplify the language used to help avoid both the confusion and mistakes that come with hypothesis testing.
.png)
The world – like people and their experiences – is constantly changing. Think back to the apple at the ice cream shop example. If you assume that apples don’t like ice cream (your alternate) and find evidence to support it, you might've found the only supporting evidence. But if you rerun the same study (when the apple doesn’t have that toothache), you’ll find evidence that actually supports the null: apples do, in fact, like ice cream.
If something is always true, finding even one negative instance is evidence to suggest otherwise. If apples always like ice cream, finding even one apple who hates ice cream will challenge this idea. What’s harder to know is if you’ve found the only evidence to support an unstable hypothesis. The relationship between apples and ice cream is more complex and might need further investigation.
Or take this example hypothesis from a digital product perspective: "For the small business segment, adding social media content to the homepage will lead to increased session durations."
You could test this hypothesis multiple times with different samples of participants. In one sample, you might notice a somewhat large duration increase while you might see a small increase in another. But if you found even one sample where session duration went down, you would have evidence to say "adding social media might not increase session durations”.
If your hypothesis was true, it should theoretically be true for every sample. You don't "accept" hypotheses because you need an infinite number of examples to prove a hypothesis, but just one counterexample to disprove it.
You need only one counterexample to disprove any hypothesis.
Keep in mind that having your hypothesis "rejected" isn't a bad thing. If anything, it's expanding how you and your stakeholders are thinking about the product or experience. If adding social media content caused session durations to drop, perhaps the relationship between variables is more complex than assumed. Or maybe the relationship doesn't actually exist at all.
Use moments when your hypothesis is "rejected" as a chance to refine hypotheses based on study results or to jump into qualitative research to identify theories and more specific hypotheses to study.
Your hypotheses give your quantitative instruments focus. You use your instruments to collect data for you. But when you test your hypotheses with this data, you realize that not all quantitative data is the same.
- Null hypothesis; alternate hypothesis
- Null hypothesis significance testing (NHST)
- Directional hypothesis
- Inverse relationship; direct or proportional relationship