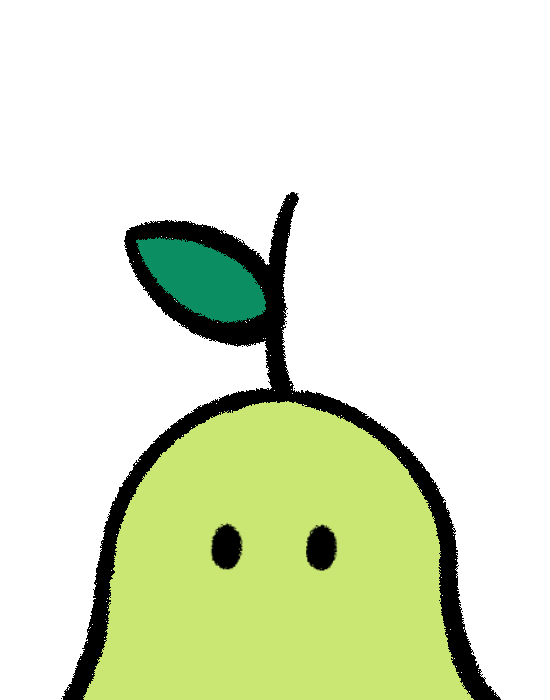

Would you rather have 10 participants or 1,000? What sounds obvious is much more complicated in practice. Having more participants can help increase your confidence in your data. And yes, small samples can be bad if they’re unrepresentative of your important segment(s), uninformative for your specific questions, and unreasonable and slow when managing, recruiting, and scheduling participants. If your sample is small by accident or without care, you’ve affirmed that small samples are indeed bad.
But if you design a study to make use of the strengths of a smaller, manageable sample, you’re able to spend far fewer resources on recruitment and deliver value to your stakeholders. So what are the strengths of a small sample?
Existence-proof
.png)
Sometimes, all it takes is one participant to say or do something for hidden patterns to emerge in your data. One participant might help your stakeholders completely reframe their understanding of the problem or recognize an untapped opportunity to make a solution. One participant might help your stakeholders say, “Oh, I never thought of that,” or “That makes sense now!”
Other times, it’s one participant who challenges a long-held but evidence-less belief your stakeholders have. If they all believe the new dark mode feature is easy for anyone to use, finding even one person who struggles with it is evidence to suggest otherwise. Think of n = 1 as existence-proof; one person is all it takes to showcase that a problem, solution, or pattern exists.
If you’ve found data that suggests this very specific problem or pattern exists, there’s always a chance that you’ve accidentally stumbled across a fluke or a natural, random anomaly. But once you know a problem or pattern exists, your next question should be around prevalence and scale.
Once you know a problem or pattern exists, your next question should be around prevalence and scale.
How many people have this problem? How many times does someone encounter this problem a week? What proportion of the population or segment reports experiencing this problem? Avoid jumping into generalized themes from one unexpected participant. Take the time to understand what patterns from one participant exist at the population level (such as with a survey to quantify themes from an interview. See Collection 4, Handbook 2 for more on surveys).
Research Velocity
When you’re dealing with small samples, you can move quickly. If your stakeholders want to test a design, a handful of participants could be enough for them to learn and make quick changes. Struggling with recruitment? 5-7 really well-structured interviews could be just enough to see value from research, making it easier to get resources for future studies.
You can also manage your own time better with smaller samples happens too. You can plan to run multiple back-to-back studies with smaller samples without feeling fatigued. You can be more thoughtful with recruitment, using scheduling emails as a way to build rapport. You can even interweave a handful of participants throughout and during your other meetings and priorities.
Plus, smaller samples won’t eat into your limited sampling frames as fast as needing hundreds – if not thousands – of participants would, meaning you can stretch a limited sampling frame farther. You can produce more knowledge faster without exhausting yourself.
Flexible Focused
Given your limited energy, you can be more selective about whom you learn from when working with smaller samples. If you want to learn from bananas who skateboard, you'd only need to recruit a few who skateboard for a study. Small samples also allow you the flexibility to follow the data.
If you find out during research you need to learn from the moms of skateboarders, you can add an additional small set of these participants to your study design. You end up saying “no” to more participants to only study the most meaningful or informative ones.
Richness vs. Generalization
As you’re reading this chapter, you might have a thought rattling around in your head: how can a handful of participants and their data be generalized to the larger population? And you’d be right in asking this question. A small sample of few people likely won’t produce generalizable learning. But that’s the point.
Small samples produce more impactful learning than any big sample ever could. As you add more participants, you turn to numbers and statistics. But after a certain point, the numbers can all blend together. How much more generalizable is the data from 150,000 participants instead of 125,000? Not to mention, your stakeholders would likely trust the findings even if it were only 1,000 participants in a well-structured study.
But with smaller samples, you can add richness to your findings (through quotes, videos, audio clips, etc.) that you can’t easily do with percentages, bar graphs and pie charts. The findings might not generalize, but your stakeholder’s perception and understanding of the problem, context, or experience can be changed by getting familiar with a set of limited people and their data.
Small samples also make it easier for your stakeholders to get involved. They can attend 2-3 remote interview sessions and take notes. If you have budget and support, you can even propose field research and get your stakeholders out of the office (check out this book for more on field research).
With smaller samples (or a small set of study locations or sites), you can help stakeholders learn directly from the people they're trying to help. They can get closer to environments they believe they understand and recognize evidence to update those beliefs. Even a few hours of field research – away from the office and the workstations – can be tremendously valuable for learning and impact.
Don’t hesitate to propose smaller samples with your qualitative, tactical, or short-term research. Explain the benefits above and work to find numbers that fit your stakeholder’s needs. But sometimes, no matter the evidence, someone will demand a large sample. Let’s now address one of the most incorrect assumptions that non-researchers hold about sample sizes: the only good sample size is a large one.
- Findings generalization; generalizability
- External validity
N/a