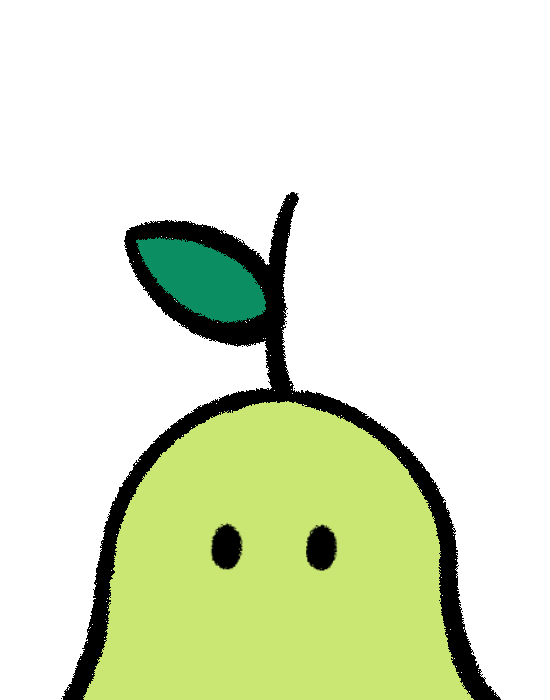

Different Qualitative Data Analysis Techniques
There are several ways to approach qualitative data analysis. Based on your study design and goals, you should collect data knowing what type of analysis you’ll need to use. Other approaches (like content, framework, or narrative analysis) are listed in search at the end of this handbook for your reference. You can read about additional qualitative data analysis techniques beyond thematic analysis here.
Make sure the analytic approach you choose matches and works with the purpose of design of your qualitative study. However, in most qualitative experience research, taking a thematic analysis approach will be best. Why is thematic analysis commonly used? It’s possibly the most “intuitive,” approachable, flexible, and inclusive approach out there.
The Thematic Analysis Process
Thematic analysis is about recognizing the themes or underlying patterns of meaning within your qualitative data. In your qualitative studies, you’re setting out to learn about how people make sense of the product/experience and the world around it. You don’t need a lot of data or a lot of experience to practice thematic analysis. As you’ll read, someone completely new to your qualitative data could recognize themes and patterns (even if they’re not as contextual as yours).
It’s an approachable QDA strategy to get started with and good at. Even if you had only one interview participant, you can still generate meaning and learn something valuable. (This is one reason why “small” sample sizes aren’t effective criticisms against qualitative research. Read more about power of “small” samples in this Topic).
The diagram below outlines the general process when using thematic analysis. You start with raw qualitative data (such as an interview or observation notes) and slowly group them to find and create themes. For arguably the best resource on thematic analysis, read this 2006 paper by qualitative researchers, Virginia Braun and Victoria Clarke. If anything, you should consider their paper essential reading for becoming a better qualitative researcher, especially the multiple, visual diagrams in the appendix.
The diagram below shows how you move from raw qualitative data (what you collect or record in your qualitative sessions) all the way to qualitative themes.
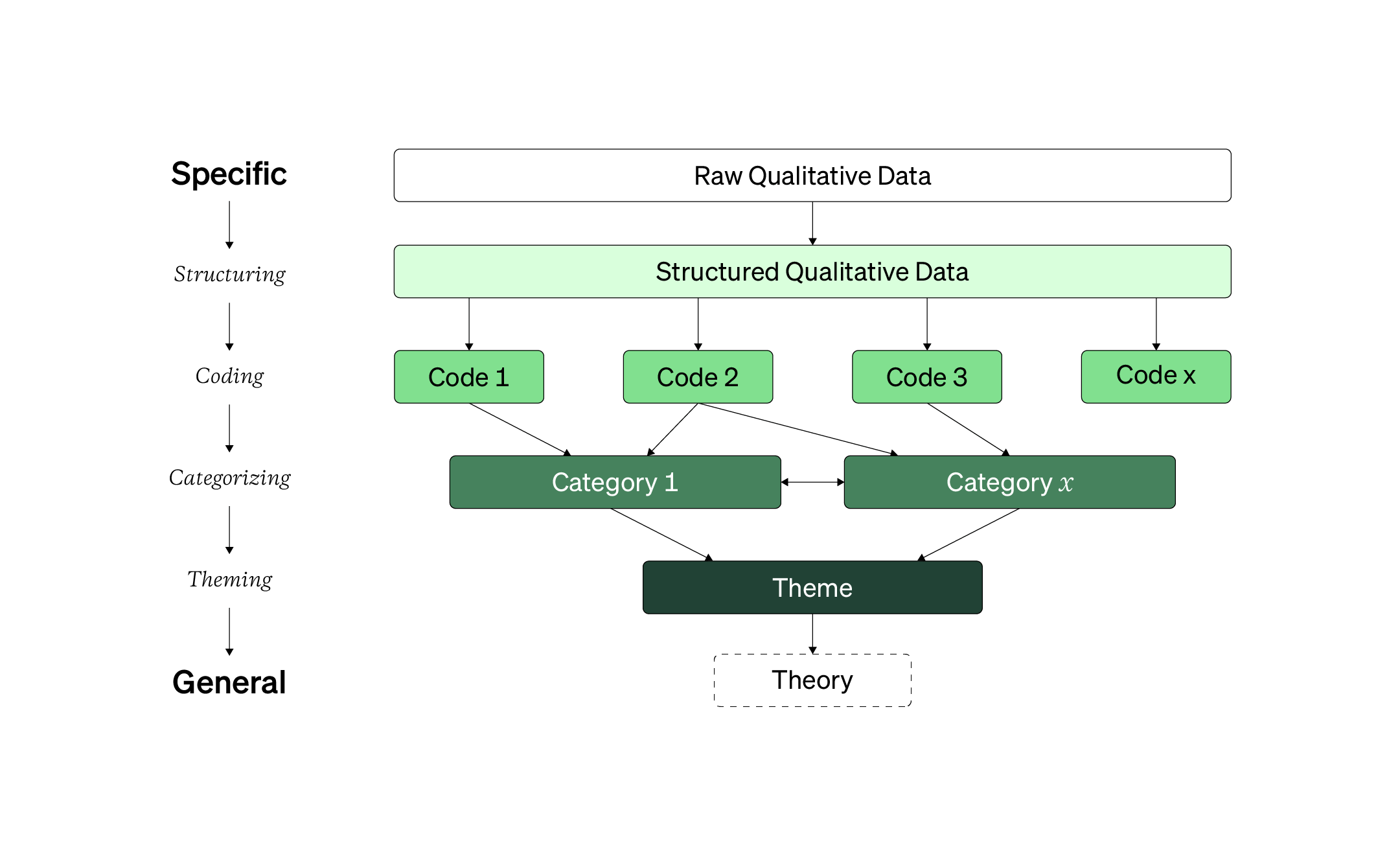
Structuring your qualitative data means taking your raw interview notes, transcripts, and more and making it easier to interact with. This might mean copy/pasting all of your interview notes into a spreadsheet tool or rearranging columns to see the data in a specific way. It’s about changing how you interact and represent the data, not about altering or distorting the raw qualitative data itself.
There aren’t guides on structuring because it’s up to you, the researcher, to figure out how best to analyze your data with the tools you have. Get scrappy and get creative with your qualitative data structuring.
Let’s break down the other steps so you can apply thematic analysis effectively.
When Qualitative Coding, Think Labels & Hashtags
The thematic analysis starts with someone known as qualitative coding. Coding is about labeling or describing the qualitative data you’ve collected. What’s there? What is someone trying to say? How can you group the various things people said or did?
Coding is about summarizing or condensing qualitative data into a short, scannable, and understandable label. This label represents the data, making it easier to refer to the labels instead of rereading large chunks of qualitative data.

It’s similar to using hashtags when you share a social media post. The hashtag helps categorize the content you’re sharing. It also gives meaning to the picture itself. If you saw a post of a veggie burger with “#Delicious,” you can see that the poster wants to let the world know they’re eating something that tastes good.
Or the person who shared the image thinks that veggie burgers are delicious. Remember that qualitative research assumes that the “truth” is created and must be interpreted. Jump back to this Topic for more.
What makes coding more complex is how you label the data. There are different ways to code your data, but to make coding approachable, think of your codes falling into two buckets: explicit or implicit codes.
Use Both Explicit and Implicit Codes
Explicit codes (or semantic codes) label what’s observable in your qualitative data. With the veggie burger example, you could use explicit codes like “#meal,” “#food,” or “#burger.” Explicit codes are objective or literal, meaning two people should use similar codes to label the same text.
Implicit codes (or latent codes), on the other hand, are based on meaning, interpretation, and perspective. This is about what this text means, not about what’s written or said. This means two people could interpret the same thing completely differently.
Some qualitative data can lead to different, even contradictory implicit codes.
In the veggie burger example, two people could order the same veggie burger but use two different hashtags to describe the burger in a social media post. The hashtags could even contradict each other (“#Delicious” vs. “#Disgusting), but they’d both be true because they’re from two different, constructed perspectives.
Your unique experiences, perspective and lens on the world are makes QDA powerful. Your lens makes it easier to recognize what someone means, behind what’s they say or do (read more about your personal lens in this Topic).
Other codes are listed below for reference, but a quick search online can show you the vast world of qualitative codes.
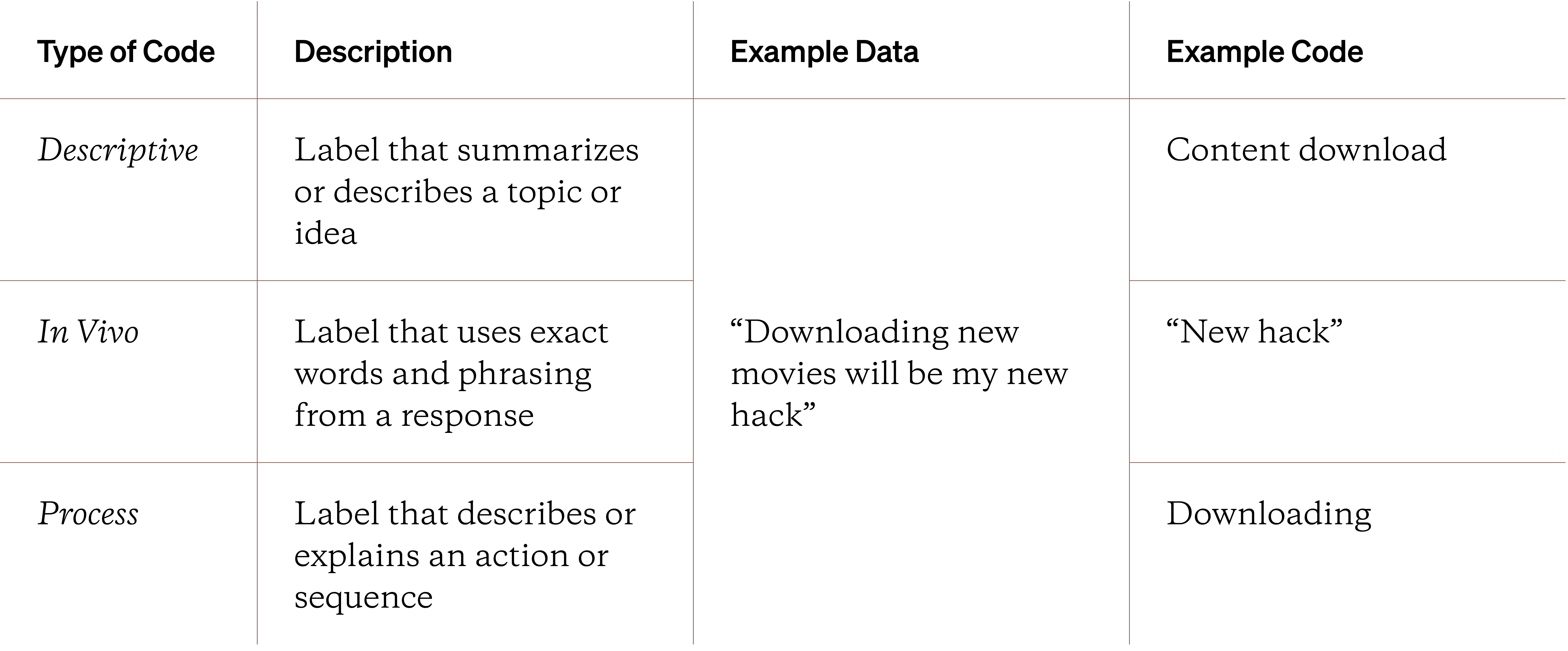
Make a Codebook
Get in the habit of making and using a codebook. This is a document or spreadsheet that lists & describes relevant codes for a specific qualitative data set. Building a codebook before starting a study is one way to speed up your qualitative data analysis. It gets even better when you have teammates help code and refine the codebook for the study.
Creating a codebook before you’re done collecting qualitative data can speed up your analysis.
Every code you make should have the following minimum elements: a code abbreviation, definition, and example. You can see an example of all three elements in a codebook used for understanding how gaming communities form.
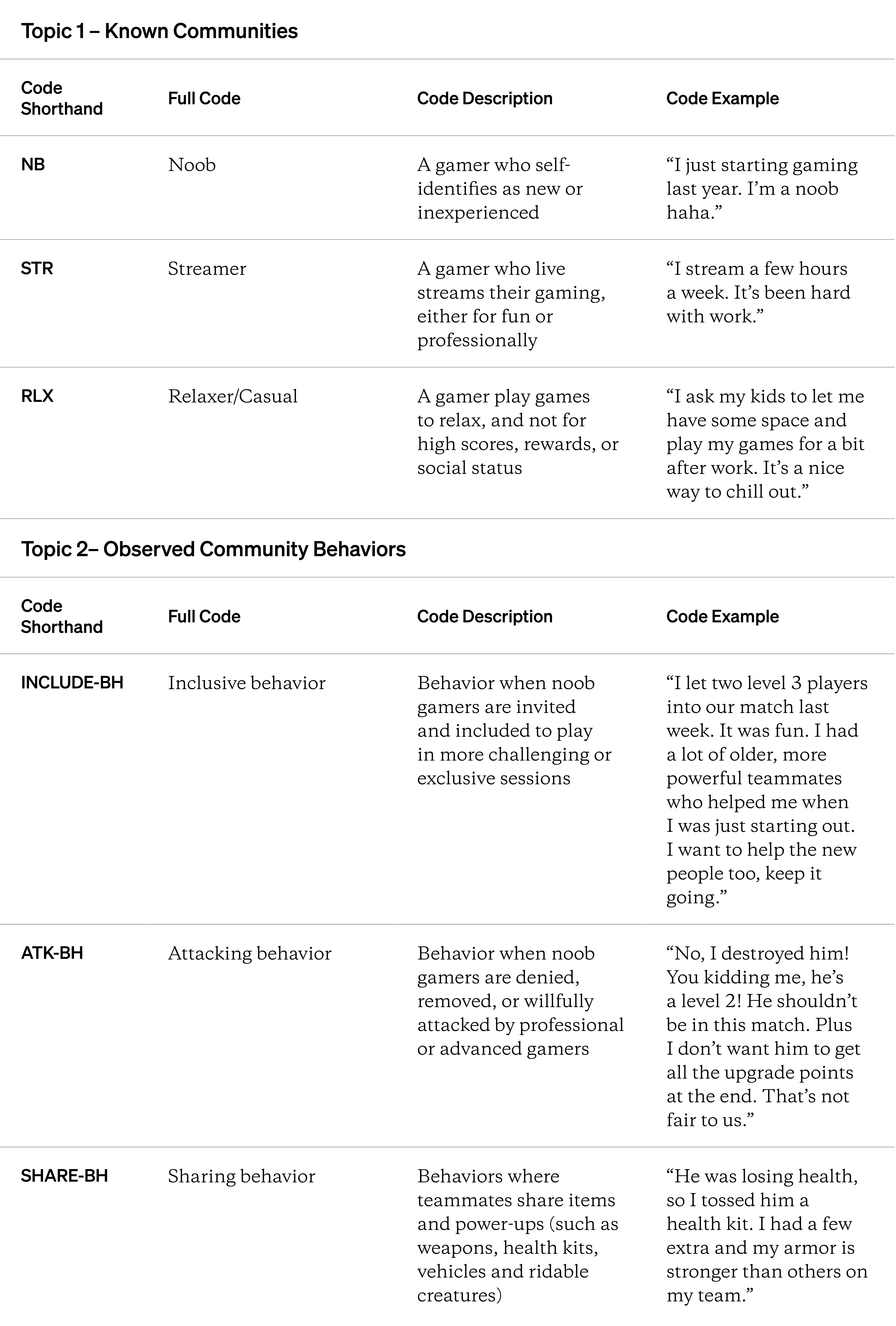
A code abbreviation is a shorthand or smaller phrase or word for a larger code. For example, it’s faster to type or write “BH” instead of “behavior”. You’d be surprised how much quicker you can code large volumes of qualitative data when you plan and use code shorthands. Remember to be consistent and avoid duplicate or similar codes (if “BH” was the shorthand for “behavior”, you shouldn’t have it also be a shorthand for “body heat”).
One of the most important things in your codebook is to describe what a code means, even if it’s an explicit code. Define in simple language what a code means. If another researcher or stakeholder wants to review your coded data, they should be able to understand why certain codes were applied to certain data chunks.
Using code definitions is also one practical way for increasing the dependability and confirmability of your qualitative research (jump to this Topic for more).
The final codebook you should have is a column for a code example. An example helps you (and latter researchers or stakeholders) have one or more tangible instances of a code being used. When you first start coding, you might need to keep this column blank. But as you revisit earlier codes and start to recognize patterns, drop in relevant examples. One tip is to add multiple examples, so you have options for selecting quotes or critical moments to share in your research findings report.
No starting codebook will be perfect; it’ll evolve as your understanding of your qualitative data deepens.
While having a codebook can be faster than just reading and coding inductively, keep in mind that might introduce bias in your analysis. You’re forcing your data to fall into specific, predetermined labels. You’re possibly missing out on understanding the variability or diversity in your data, and you might wind up confirming what you’ve expected.
No codebook is perfect so expect it to change throughout your QDA process. Codebook changes are good signs you’re getting away from your expectations and closer to the meaning within the data.
First, Code for Volume
You can code the same text with multiple codes or choose not to code something. When first analyzing your data, you want to code freely and for quantity. When you revisit the codes, you can cut them, combine them, rename them, and more. But always start your analysis by coding as much of your data as possible to recognize patterns that aren’t readily obvious.
.png)
Make sure you’re not “cherry-picking” what you do code. This means you overly focus on qualitative data that’s (1) vivid, dramatic, or surprising or (2) segments that only support what the team already wants to do or expects to learn. Coding this way can lead to sensational but skewed findings.
Be cautious about the importance you give those segments relative to other “uninteresting” segments. When you go to test your themes (the result of your coding), you’ll see how poorly they fit the larger, “uninteresting segments.
Then, Combine/Delete/Update/Rename Codes
The second time you read your qualitative data after your initial wave of codes, you’ll notice patterns. Codes that made sense on the first read-through now exist as part of a larger group of similar codes.
Don’t be afraid to rename and cut codes. The purpose of coding is to make it easy to extract meaning from your qualitative data, not to tire yourself out by coding every single word or sentence.
Having more codes doesn’t translate to better qualitative data analysis.
Not every code will be important or even relevant when you reread them. It can be helpful to move some codes into their own “temporary” group. Near the end stages of your coding, you can review these codes to see they add weight to the relevant, meaningful codes you have or if they challenge those codes. You’ll have to make a decision to rename or relabel, combine with other codes, or remove all the “temporary” codes completely.
It’s important to know that qualitative coding - especially for new/inexperienced researchers or stakeholders - can be tiring.
Coding can be Fatiguing
Qualitative coding requires an incredible amount of attention and patience. It’ll take energy to recognize and describe the patterns you see in your qualitative data. And rereading the same text repeatedly can feel tedious. But recognize that coding is a skill.
The more you code, the faster you become.
The more you practicing coding and the more experience you have with relevant topics and populations, the faster and more credibly you’ll code. If you spent one full-year coding and working with qualitative data, you’ll be surprised how quickly and efficiently your coding abilities will become. Below are some additional tips to avoid coding fatigue as much as possible.
Strategies to Avoid Coding Fatigue
- Use the find/replace feature whenever you can (especially when renaming codes or by expanding code abbreviations)
- Schedule or budget time to code between meetings and other priorities
- Code in chunks (such as 45 minutes of coding, then a 5 minute break, and then 10 minutes rereading all the qualitative data and codes)
- Doodle or draw relationships between codes to avoid getting burnt out
- Code with your stakeholders or other researchers, whenever possible
- Create a starting codebook when you’re finalizing your qualitative study design or method
- Reduce friction and busywork as much as possible (aka don’t write down qualitative data onto Post-its first)
After you’ve coded all of your data, you then start forming categories and creating themes.
- Grounded theory
- Narrative analysis
- Framework analysis
- Conversation analysis
- Discourse analysis
- Concept-driven/deductive vs. data-driven/inductive coding approaches
- RQDA (qualitative data analysis using the R programming language)